Motor learning tasks are often categorized into motor adaptation, which learns recalibration of existing motor policies to perturbed environment, and motor skill learning, which learns novel motor policies to achieve task goals. Learning to walk on the moon would be a good example of motor adaptation. On the moon, where gravity reduces to sixteen percent on the earth, you need to recalibrate your existing working patterns. For the motor skill learning, you can think of playing the piano. You need to learn new patterns of finger movements and how to distribute force on your fingers to play musical notes.
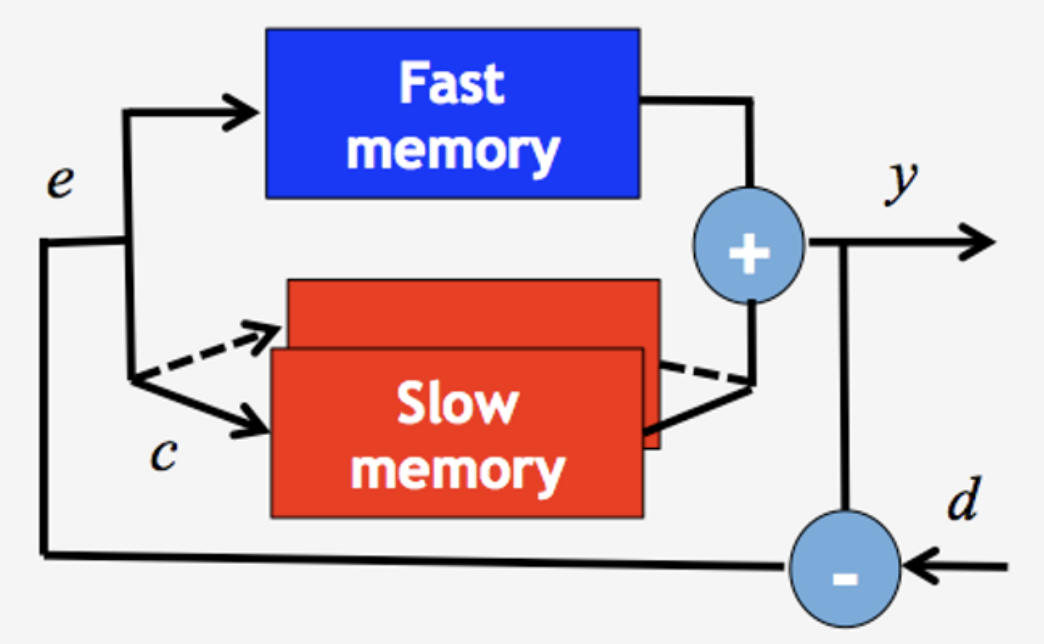
Motor learning can be quantified easier than other types of learning, such as word learning and abstract-rule learning. Changes of reaction time, movement speed and direction, force could be measured as indicators of learning. Thus, motor learning is very attractive in the perspective of theoretical computation modeling. Our lab pursues to use computational models as tools to understand human behaviors and underlying neural mechanisms in motor adaptation and motor skill learning. In this blog, I will focus on motor adaptation, please see the other blog linked to brain-machine interface for motor skill learning.
For motor adaptation models, we employed well-known multi-rate memory models as depicted in the figure below.
According to this model, motor output (y) is represented as the sum of two memory states with different time scales, fast memory and slow memory.
Each memory updates its state proportional to motor errors (e), which is the difference between the desired motor output (d) and the actual motor output (y). Fast memory updates its state from the current trial to the next trial faster and decays faster than slow memory. Additionally, one-fast and multiple slow memory could account for learning multiple tasks; initial interference in fast memory with gradual learning of separate representation of multiple tasks in distinct slow memory. A switching cue (c) plays a role in addressing corresponding slow memory depending on a current task.
In recently published papers in PLoS One (2015), we showed how this simple computational model could account for contextual interference effects and spacing effects. Based on this model, we also searched neural substrates of motor memory with multiple time scales spatially distributed in the brain (PLoS Biology, 2015) as shown in the following figure. (https://www.youtube.com/watch?v=1tc98aBU67w)

Currently, we investigate on interaction between motor memory and episodic memory, which are two different form of memory, when two relevant tasks were sequentially presented. We found preliminary results showing bidirectional interference between the two memories not only in behaviors but also in neural activities using fMRI.
In the future, we will further investigate causal link of this interference using non-invasive brain stimulation using transcranial magnetic stimulation (TMS).
#motorlearning #computationalmodel #motoradaptation #fmri